Create and manage Relevance Generative Answering (RGA) models
Create and manage Relevance Generative Answering (RGA) models
|
Coveo Relevance Generative Answering (RGA) is a paid product extension. Contact Coveo Sales or your Account Manager to add RGA to your organization license. |
Relevance Generative Answering (RGA) leverages generative AI technology to generate answers to complex natural language user queries in a Coveo-powered search interface.
What does an RGA model do?
When an RGA model builds, it creates embeddings for the indexed items specified in the model settings and stores the embeddings in model memory.
|
Note
The model is preconfigured to rebuild and update the embeddings weekly based on when the model is created. Contact your Coveo Account Manager if a different build interval is required. |
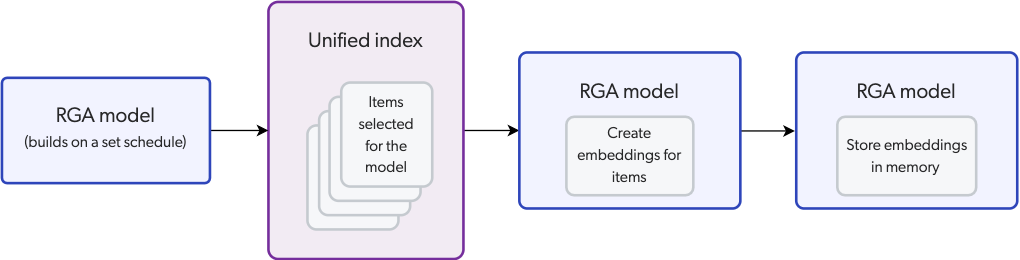
An RGA model uses a pre-trained sentence transformer language model to create the embeddings. The language model does this by capturing relationships between words, phrases, and sentences in the dataset.
An RGA model creates embeddings only for the content in an item’s body, which is the content mapped to the body
field in the Coveo index.
For more information, see How RGA uses your content.
As shown in the following diagram, the model uses chunks to create the embeddings. Instead of creating a vector for each individual word, a vector is created for a segment of text (chunk) to increase relevance.
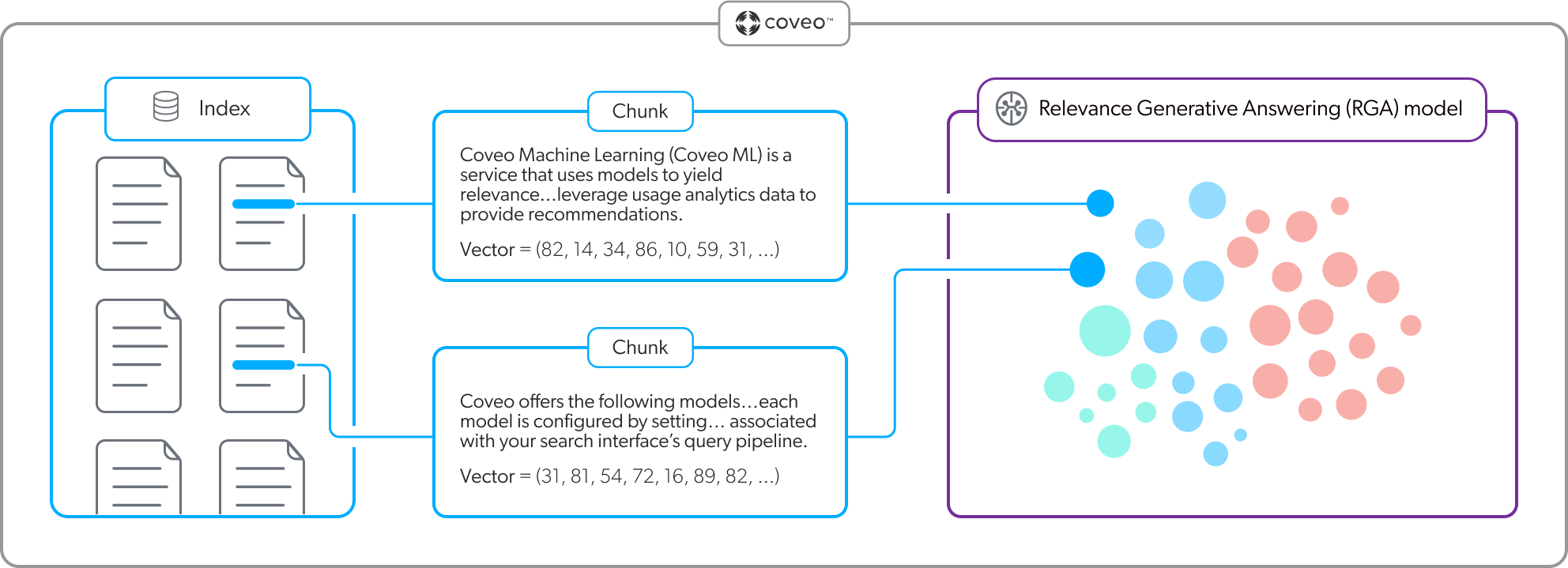
As shown in the following diagram, when a user enters a query in a Coveo-powered search interface, the RGA model embeds the query in the embedding vector space in its memory to find the most relevant segments of text (chunks).
In the context of the RGA answer-generation flow, this is referred to as second-stage content retrieval. Only the chunks corresponding to the most relevant items identified during first-stage content retrieval (items retrieved by the Coveo search engine) are considered. The most relevant chunks are then used to generate an answer.
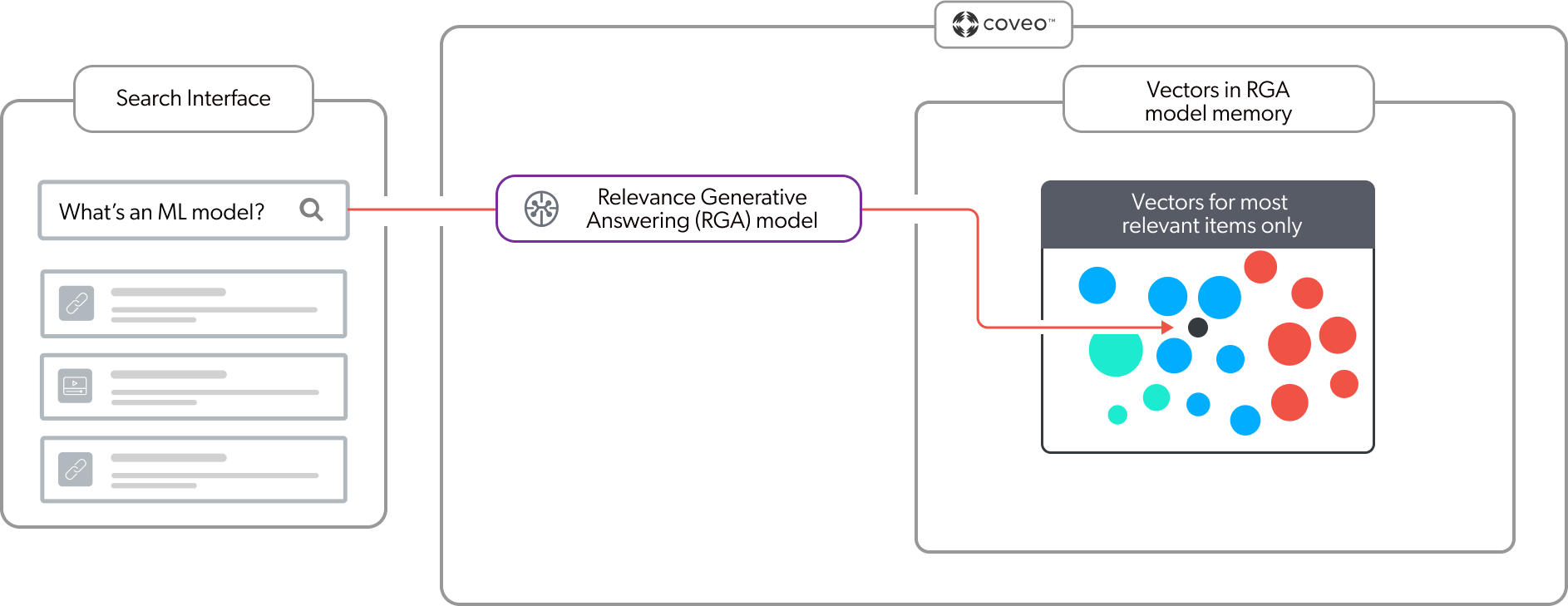
|
The embeddings that are created by the RGA model aren’t impacted by usage analytics events. However, an RGA model leverages the most relevant items retrieved by your Coveo-powered search for a given user query. Therefore, by enabling an Automatic Relevance Tuning (ART) model, which learns from usage analytics events, then the most relevant items, and by extension the generated answer, will be influenced by usage analytics events. |
|
Note
You can set a custom value for the maximum number of items that the RGA model considers when retrieving the most relevant segments of text (chunks). You can also modify the relevancy threshold that’s used by the RGA model to determine if a chunk is relevant enough to be considered by the RGA model for answer generation. These are advanced model query pipeline association configurations that should be used by experienced Coveo administrators only. For more information, see RGA model association advanced configuration. |
Prerequisites
-
You have the required privileges to create an RGA model.
-
The content you want to use for the model meets the item requirements and is optimized for RGA.
NoteAn optimal RGA implementation includes both an RGA model and a Semantic Encoder (SE) model. The same SE model can be used with multiple RGA models. For best results, both models should be configured to use the same content.
See RGA overview for information on how RGA and SE work together in the context of a search session to generate answers.
Keep the model embedding limits in mind when choosing the content for your model.
Create an RGA model
-
Depending on whether models have already been created in your Coveo organization:
-
If your Coveo organization doesn’t contain any models, on the Models (platform-ca | platform-eu | platform-au) page, click the Relevance Generative Answering card.
-
If your Coveo organization already contains models, on the Models (platform-ca | platform-eu | platform-au) page, click Add model, and then click the Relevance Generative Answering card.
-
-
Click Next.
-
In the Learn from section, select the content that the model will use. You can select the sources and apply additional filters using the Standard configuration, or use Advanced mode to define a custom filter expression.
You’ll lose the current mode settings when you switch between Standard and Advanced mode.
NoteAn optimal RGA implementation includes both an RGA model and a Semantic Encoder (SE) model. The same SE model can be used with multiple RGA models. For best results, both models should be configured to use the same content.
See RGA overview for information on how RGA and SE work together in the context of a search session to generate answers.
The Data volume preview section shows the impact of your settings on the data that’s available to the model.
-
In the Standard tab:
-
In the Sources dropdown menu, select the sources that contain the items from which you want the model to learn.
NoteIf your Coveo organization includes multiple indexes, the model can learn only from sources that are linked to the default index.
-
(Optional) In the Apply filters on dataset section, you can specify a condition to segment the content on which the model should base its training:
ExampleYou want the model to base its training only on items for which the collection field have the
FAQ
value.Therefore, you add a
collection is equal to FAQ
condition.-
Click Add filter(s).
-
In the Field name input, enter the name of the field that you want to use to segment the dataset.
-
In the Select an operator dropdown menu, select the desired operator.
-
In the Value input, enter the value of the field on which you want to segment the dataset.
-
Click Apply.
-
-
-
In the Advanced tab:
-
Enter a custom filter expression using Coveo query syntax.
-
Click Apply.
-
-
-
Click Next.
-
In the Name your model input, enter a meaningful display name for the model.
-
Use the Project selector to associate your RGA model with one or more projects.
-
Click Start building.
-
You can then associate the model with a pipeline to take advantage of the model in a search interface.
Edit an RGA model
-
On the Models (platform-ca | platform-eu | platform-au) page, click the model you want to edit, and then click Edit in the Action bar.
-
On the subpage that opens, select the Configuration tab.
-
In the upper-right corner, click Edit.
-
Under Name, edit the model’s display name.
-
Use the Project selector to associate your RGA model with one or more projects.
-
In the Learn from section, select the content that the model will use. You can select the source(s) and apply additional filters using the Standard configuration, or use Advanced mode to define a custom filter expression.
You’ll lose the current mode settings when you switch between Standard and Advanced mode.
NoteAn optimal RGA implementation includes both an RGA model and a Semantic Encoder (SE) model. The same SE model can be used with multiple RGA models. For best results, both models should be configured to use the same content.
See RGA overview for information on how RGA and SE work together in the context of a search session to generate answers.
The Data volume preview section shows the impact of your settings on the data that’s available to the model.
-
In the Standard tab:
-
In the Sources dropdown menu, select the sources that contain the items from which you want the model to learn.
NoteIf your Coveo organization includes multiple indexes, the model can learn only from sources that are linked to the default index.
-
(Optional) In the Apply filters on dataset section, you can specify a condition to segment the content on which the model should base its training:
ExampleYou want the model to base its training only on items for which the collection field have the
FAQ
value.Therefore, you add a
collection is equal to FAQ
condition.-
Click Add filter(s).
-
In the Field name input, enter the name of the field that you want to use to segment the dataset.
-
In the Select an operator dropdown menu, select the desired operator.
-
In the Value input, enter the value of the field on which you want to segment the dataset.
-
Click Apply.
-
-
-
In the Advanced tab:
-
Enter a custom filter expression using Coveo query syntax.
-
Click Apply.
-
-
-
Click Save.
Delete an RGA model
|
Note
Models are automatically dissociated from all their associated query pipelines once they’re deleted. |
-
On the Models (platform-ca | platform-eu | platform-au) page, click the ML model that you want to delete, and then click More > Delete in the Action bar.
-
In the Delete a model panel that appears, click Delete Model.
Review active model information
On the Models (platform-ca | platform-eu | platform-au) page, click the desired model (must be Active), and then click Open in the Action bar (see Reviewing model information).
Reference
Model embedding limits
The RGA model converts your content’s body text into numerical representations (vectors) in a process called embedding. It does this by breaking the text up into smaller segments called chunks, and each chunk is mapped as a distinct vector. For more information, see Embeddings.
Due to the amount of processing required for embeddings, the model is subject to the following embedding limits:
|
Note
The same chunking strategy is used for all sources and item types. |
-
Up to 5 million items or 50 million chunks
Notes-
The maximum number of items depends on the item allocation of your product plan.
-
Coveo strongly recommends that you add a Semantic Encoder (SE) model as part of your RGA implementation. If you have more than one RGA model in your Coveo organization, for best results each RGA model should use only the items that are used by the SE model.
-
-
1000 chunks per item
This means that for a given item, there can be a maximum of 1000 chunks. So if an item is long with a lot of text, such as more than 200,000 words or 250 pages, the model will embed the item’s text until the 1000-chunk limit is reached. The remaining text won’t be embedded and therefore won’t be used by the model.
-
250 words per chunk
NoteThere can be an overlap of up to 10% between chunks. In other words, the last 10% of the previous chunk can be the first 10% of the next chunk.
"Status" column
On the Models (platform-ca | platform-eu | platform-au) page of the Administration Console, the Status column indicates the current state of your Coveo ML models.
The following table lists the possible model statuses and their definitions:
Status | Definition | Status icon |
---|---|---|
Active |
The model is active and available. |
|
Build in progress |
The model is currently building. |
|
Inactive |
The model isn’t ready to be queried, such as when a model was recently created or the organization is offline. |
|
Limited |
Build issues exist that may affect model performance. |
|
Soon to be archived |
The model will soon be archived because it hasn’t been queried for an extended period of time. |
|
Error |
An error prevented the model from being built successfully. |
|
Archived |
The model was archived because it hasn’t been queried for at least 30 days. |
Required privileges
By default, members with the required privileges can view and edit elements of the Models (platform-ca | platform-eu | platform-au) page.
The following table indicates the privileges required for members to manage Coveo Generic models (see Manage privileges and Privilege reference).
Action | Service - Domain | Required access level |
---|---|---|
View models |
Machine Learning - Models |
View |
Manage models |
Organization - Organization |
View |
Machine Learning - Models |
Edit |
|
Machine Learning - Allow content preview |
Enable |
|
Content - Sources |
View All |
|
Content - Fields |
View |