About the Coveo Relevance Maturity Model
About the Coveo Relevance Maturity Model
The Coveo Relevance Maturity Model™ (CRMM) can help you visualize your journey towards search relevance:
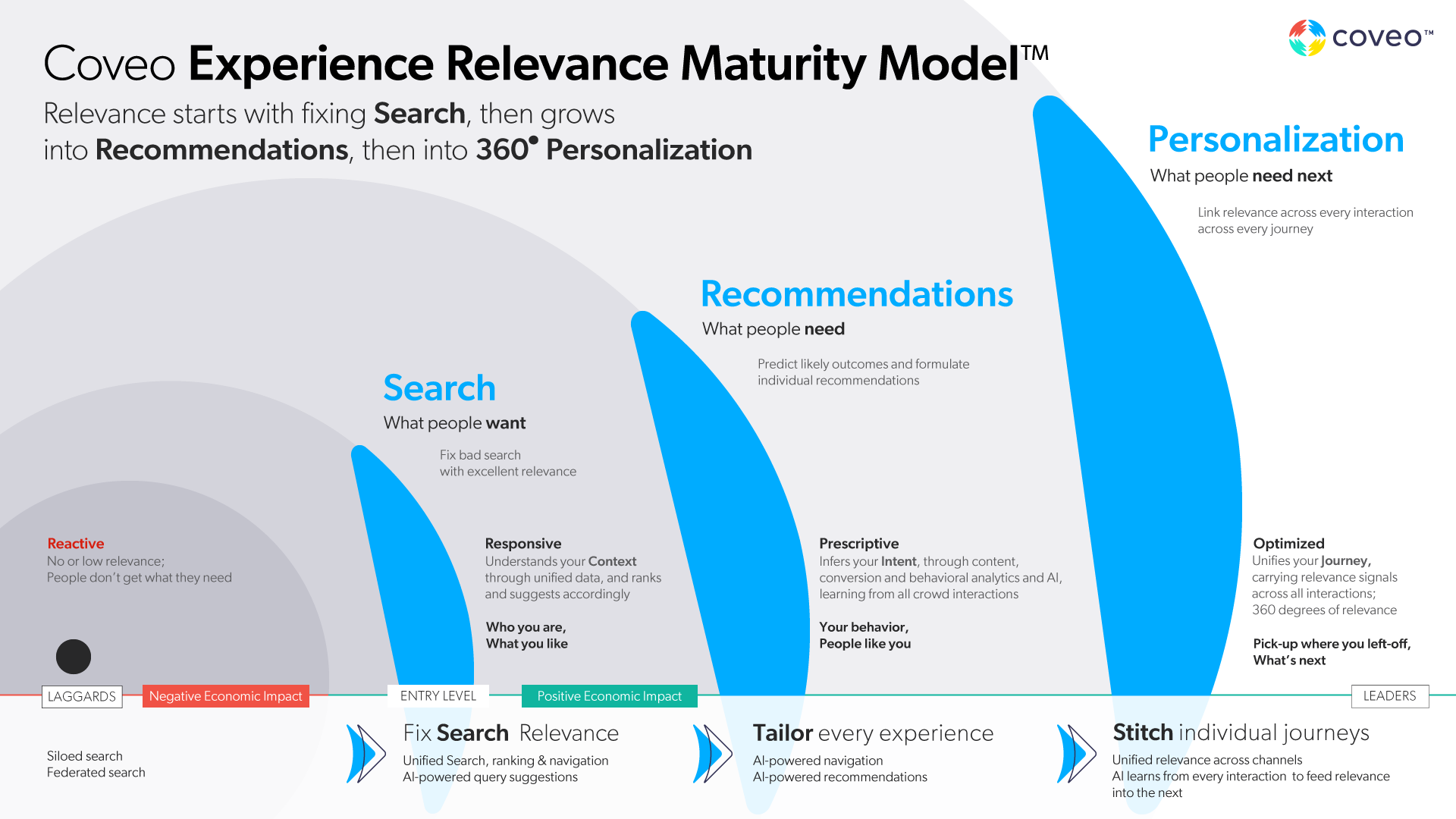
Reactive search
Reactive search could be considered the opposite of relevance. Think of it as asking your friends a question. They may or may not know the answer. Once you have all of their answers, how do you know which one is the best for you? This is what happens when you do either siloed or federated search.
As you’ll see, neither of these apply to the Coveo Platform. Our relevance engine can integrate with an unlimited number of data sources so that you can search across all of them for the most pertinent information.
Siloed search
Siloed search solutions only offer single source search, which constrains users to access each silo of information separately to achieve their search tasks. In addition, a siloed search doesn’t predict what users are searching for. Therefore, users have to explicitly request what they need to obtain quality results.
Federated search
Federated search solutions allow users to access many sources of data to find the information they need. Even if this kind of solution interrogates several data sources, it fails to rank the search results according to relevance. This can be frustrating when facing a vast information ecosystem since relevant items can appear at the bottom of the search result list.
Responsive search
Being responsive — giving people what they’ve actually asked for — is the first step in relevance maturity. Responsive search doesn’t just match keywords with content; it seeks to solve the searcher’s need.
Like federated search solutions, search results in the Coveo Platform are consolidated from many sources. However, the Coveo Platform unifies and ranks these search results according to the sources’ access controls and follows security implementations (see Coveo management of security identities and item permissions).
Unified ranking ensures the most relevant results surface at the top by applying a ranking process that filters irrelevant items.
With the Coveo Headless and Atomic libraries, you can conveniently customize your search interface to meet your users’ needs.
Prescriptive search
At this stage, you have started providing information to your users based on context. You’re also gathering insights on the way they behave with your search solution. By feeding user behavioral data to your Coveo Machine Learning (Coveo ML) models, you can further refine relevance.
Based on the data you analyzed from your users’ behavior, you will likely discover areas that may need improvement in your search solution. To overcome this lack of relevance, you can use the Query Pipelines (platform-ca | platform-eu | platform-au) page of the Coveo Administration Console, from which you can manually optimize your search results relevance and your search experience.
This powerful tool lets you modify your end-user queries without having to use any code. From the Coveo Administration Console, you can create rules to improve the relevance of your search solution such as boosting specific items and creating synonyms (see Set up a query pipeline).
Optimized search
At this stage, Coveo ML helps you to proactively act on your users’ queries by suggesting items in the result list based on the similarity of context.
Any manual tuning you do to improve your search experience is further refined by receiving additional contextual inputs at the point of the search query (see About Automatic Relevance Tuning (ART)).
The model now uses the search behavior of other users to predict the intent of a specific user by providing them with immediately relevant query suggestions (see About Query Suggestions (QS), About Smart Snippets, and How does Intelligent Term Detection (ITD) work?).
Now you can deliver an effortless search experience by providing your end users with key elements they need at a given moment, without them having to send further queries.
Coveo ML uses search navigation data to anticipate what a specific user will need next to accomplish their tasks by providing content recommendations, product recommendations, and relevant case classifications.
What’s next?
The Search project overview article outlines the steps to conduct a successful search project.